本篇是深度强化学习动手系列文章,自MyEncyclopedia公众号文章深度强化学习之:DQN训练超级玛丽闯关发布后收到不少关注和反馈,这一期,让我们实现目前主流深度强化学习算法PPO来打另一个红白机经典游戏1942。
相关文章链接如下:
NES 1942 环境安装
红白机游戏环境可以由OpenAI Retro来模拟,OpenAI Retro还在 Gym 集成了其他的经典游戏环境,包括Atari 2600,GBA,SNES等。
不过,受到版权原因,除了一些基本的rom,大部分游戏需要自行获取rom。
环境准备部分相关代码如下
1 | pip install gym-retro |
1 | python -m retro.import /path/to/your/ROMs/directory/ |
OpenAI Gym 输入动作类型
在创建 retro 环境时,可以在retro.make中通过参数use_restricted_actions指定 action space,即按键的配置。
1 | env = retro.make(game='1942-Nes', use_restricted_actions=retro.Actions.FILTERED) |
可选参数如下,FILTERED,DISCRETE和MULTI_DISCRETE 都可以指定过滤的动作,过滤动作需要通过配置文件加载。
1 | class Actions(Enum): |
DISCRETE和MULTI_DISCRETE 是 Gym 里的 Action概念,它们的基类都是gym.spaces.Space,可以通过 sample()方法采样,下面具体一一介绍。
- Discrete:对应一维离散空间,例如,Discrete(n=4) 表示 [0, 3] 范围的整数。
1 | from gym.spaces import Discrete |
输出是
1 | 3 |
- Box:对应多维连续空间,每一维的范围可以用 [low,high] 指定。 举例,Box(low=-1.0, high=2, shape=(3, 4,), dtype=np.float32) 表示 shape 是 [3, 4],每个范围在 [-1, 2] 的float32型 tensor。
1 | from gym.spaces import Box |
输出是
1 | [[-0.7538084 0.96901214 0.38641307 -0.05045208] |
- MultiBinary: 0或1的多维离散空间。例如,MultiBinary([3,2]) 表示 shape
是3x2的0或1的tensor。
1
2
3from gym.spaces import MultiBinary
space = MultiBinary([3,2])
print(space.sample())
输出是
1 | [[1 0] |
- MultiDiscrete:多维整型离散空间。例如,MultiDiscrete([5,2,2]) 表示三维Discrete空间,第一维范围在 [0-4],第二,三维范围在[0-1]。
1 | from gym.spaces import MultiDiscrete |
输出是
1 | [2 1 0] |
- Tuple:组合成 tuple 复合空间。举例来说,可以将 Box,Discrete,Discrete组成tuple 空间:Tuple(spaces=(Box(low=-1.0, high=1.0, shape=(3,), dtype=np.float32), Discrete(n=3), Discrete(n=2)))
1 | from gym.spaces import * |
输出是
1 | (array([ 0.22640526, 0.75286865, -0.6309239 ], dtype=float32), 0, 1) |
- Dict:组合成有名字的复合空间。例如,Dict({'position':Discrete(2),
'velocity':Discrete(3)})
1
2
3from gym.spaces import *
space = Dict({'position':Discrete(2), 'velocity':Discrete(3)})
print(space.sample())
输出是
1 | OrderedDict([('position', 1), ('velocity', 1)]) |
NES 1942 动作空间配置
了解了 gym/retro 的动作空间,我们来看看1942的默认动作空间
1 | env = retro.make(game='1942-Nes') |
1 | The size of action is: (9,) |
表示有9个 Discrete 动作,包括 start, select这些控制键。
从训练1942角度来说,我们希望指定最少的有效动作取得最好的成绩。根据经验,我们知道这个游戏最重要的键是4个方向加上 fire 键。限定游戏动作空间,官方的做法是在创建游戏环境时,指定预先生成的动作输入配置文件。但是这个方式相对麻烦,我们采用了直接指定按键的二进制表示来达到同样的目的,此时,需要设置 use_restricted_actions=retro.Actions.FILTERED。
下面的代码限制了6种按键,并随机play。
1 | action_list = [ |
来看看其游戏效果,全随机死的还是比较快。
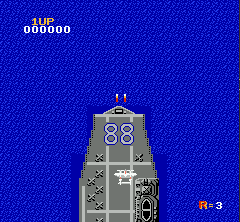
图像输入处理
一般对于通过屏幕像素作为输入的RL end-to-end训练来说,对图像做预处理很关键。因为原始图像较大,一方面我们希望能尽量压缩图像到比较小的tensor,另一方面又要保证关键信息不丢失,比如子弹的图像不能因为图片缩小而消失。另外的一个通用技巧是将多个连续的frame合并起来组成立体的frame,这样可以有效表示连贯动作。
下面的代码通过 pipeline 将游戏每帧原始图像从shape (224, 240, 3) 转换成 (4, 84, 84),也就是原始的 width=224,height=240,rgb=3转换成 width=84,height=240,stack_size=4的黑白图像。具体 pipeline为
MaxAndSkipEnv:每两帧过滤一帧图像,减少数据量。
FrameDownSample:down sample 图像到指定小分辨率 84x84,并从彩色降到黑白。
FrameBuffer:合并连续的4帧,形成 (4, 84, 84) 的图像输入
1 | def build_env(): |
观察图像维度变换
1 | env = retro.make(game='1942-Nes', use_restricted_actions=retro.Actions.FILTERED) |
确保shape 从 (224, 240, 3) 转换成 (4, 84, 84)
1 | Initial shape: (224, 240, 3) |
FrameDownSample实现如下,我们使用了 cv2 类库来完成黑白化和图像缩放
1 | class FrameDownSample(ObservationWrapper): |
MaxAndSkipEnv,每两帧过滤一帧
1 | class MaxAndSkipEnv(Wrapper): |
FrameBuffer,将最近的4帧合并起来
1 | class FrameBuffer(ObservationWrapper): |
最后,visualize 处理后的图像,同样还是在随机play中,确保关键信息不丢失
1 | def random_play_preprocessed(env, action_list, sleep_seconds=0.01): |
matplotlib 动画输出
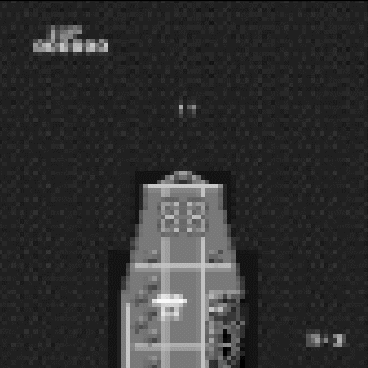
CNN Actor & Critic
Actor 和 Critic 模型相同,输入是 (4, 84, 84) 的图像,输出是 [0, 5] 的action index。
1 | class Actor(nn.Module): |
PPO核心代码
先计算 \(r_t(\theta)\),这里采用了一个技巧,对 \(\pi_\theta\) 取 log,相减再取 exp,这样可以增强数值稳定性。
1 | dist = self.actor_net(state) |
surr1 对应PPO论文中的 \(L^{CPI}\)
然后计算 surr2,对应 \(L^{CLIP}\) 中的 clip 部分,clip可以由 torch.clamp 函数实现。\(L^{CLIP}\) 则对应 actor_loss。
1 | surr2 = torch.clamp(ratio, 1.0 - self.clip_param, 1.0 + self.clip_param) * advantage |
最后,计算总的 loss \(L_t^{CLIP+VF+S}\),包括 actor_loss,critic_loss 和 policy的 entropy。
1 | entropy = dist.entropy().mean() |
上述完整代码如下
1 | for _ in range(self.ppo_epoch): |
补充一下 GAE 的计算,advantage 根据公式
可以转换成如下代码
1 | def compute_gae(self, next_value): |
外层 Training 代码
外层调用代码基于随机 play 的逻辑,agent.act()封装了采样和 forward prop,agent.step() 则封装了 backprop 和参数学习迭代的逻辑。
1 | for i_episode in range(start_epoch + 1, n_episodes + 1): |
训练结果
让我们来看看学习的效果吧,注意我们的飞机学到了一些关键的技巧,躲避子弹;飞到角落尽快击毙敌机;一定程度预测敌机出现的位置并预先走到位置。
评论
shortname
for Disqus. Please set it in_config.yml
.